Ready to achieve real impact with Data & Ai?
USE CASES
Dynamic Pricing
Helping a retail bank to increase conversion rates while maximising profit margins
Situation
In the current credit market, high competitive pressure and growing costs lead to decreasing margins with a decreasing customer base. Banks traditionally priced consumer loans based on risk costs, factoring in default risks in combination with discounts and promotional interest rates, which resulted in complex pricing system that could be difficult to manage.
Challenge
Pricing decisions relied mostly on gut feeling and experience, as their effects on demand and profitability are impossible to forecast.
In the current inflationary market environment, banks face additional margin pressure, having to balance both increasing lending costs and the willingness-to-pay of customers.
Solution
In close collaboration with the banks internal pricing team, NEWNOW developed a dynamic pricing model to regularly derive optimal credit prices differentiated for the banks customer clusters to achieve profit and volume targets.
The custom model was tailored to the unique data structure and pricing methodology of the client. NEWNOW delivered a pricing decision cockpit for the client to use for planning pricing decision scenarios with their respective impact on profitability, updating input parameters and uploading new input data.
NEWNOW combined both statistical and Machine Learning approaches to determine the demand elasticity of each customer cluster to understand their reaction to price changes.
These elasticities were then fed into an optimisation algorithm, which maximises demand by selecting a set of optimised interest rates that satisfy a given profit margin target.
An exemplary preview of the pricing cockpit solution
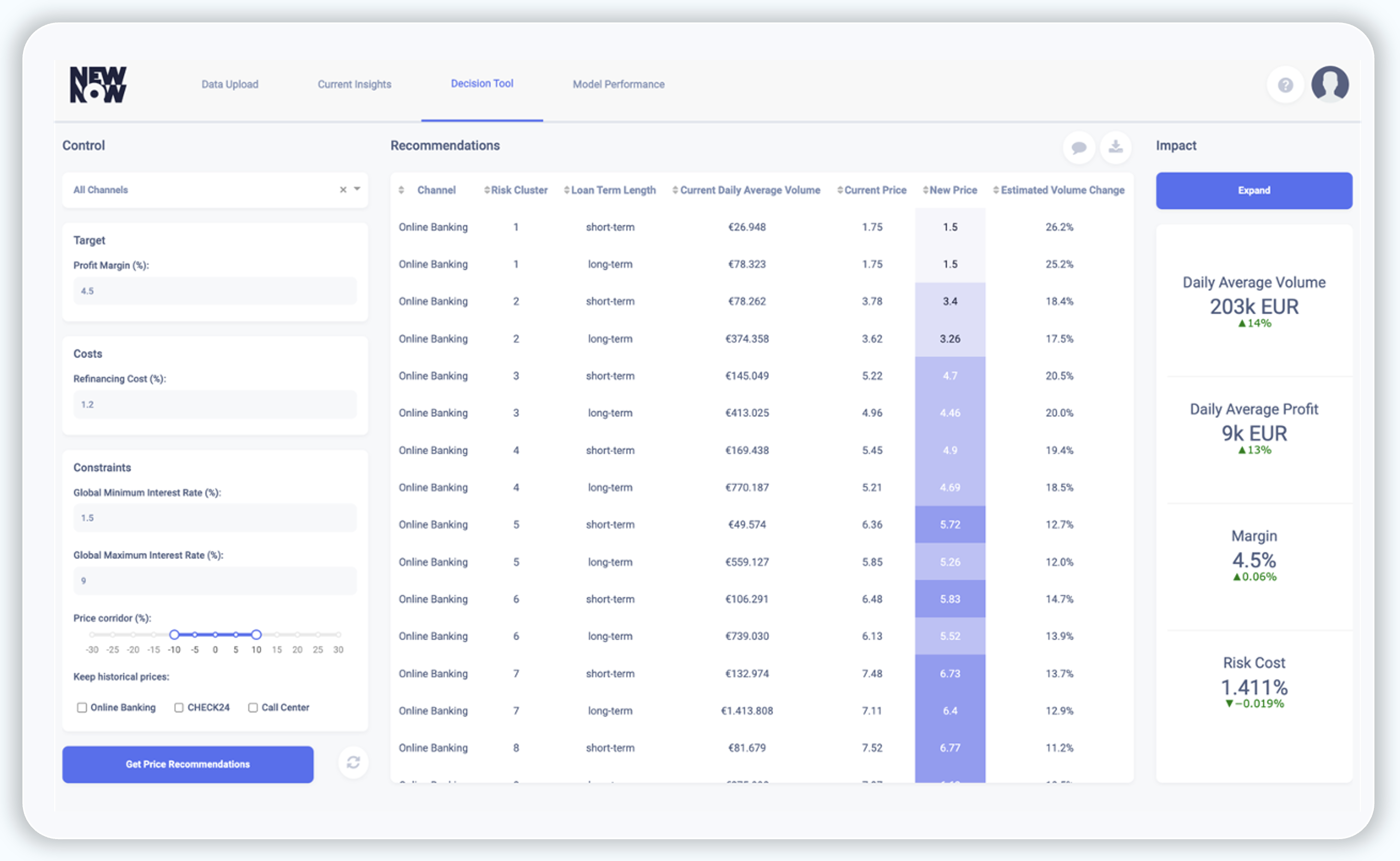
Main features of the decision cockpit:
- Control: Adjust parameters such as the target profit margin and interest rate constraints
- Recommendations: Download a set of optimised prices across different customer segments
- Impact: Explore how changing different parameters will affect performance
- Other Features: Upload and inspect new data, view visualisations and trends of your current data and inspect model performance in a simple and intuitive way
Impact
To implement our solution, we first carried out a field A/B test, where several customer clusters received optimised prices according to the model's recommendation, while other clusters received existing prices. The A/B tests showed an incremental 1,5% profit margin via optimised pricing, while achieving a 30-40% volume increase.